Background
Image reconstruction arises in many fields such as medical and pre-clinical imaging (e.g. positron emission tomography (PET), single photon emission computed tomography (SPECT), magnetic resonance imaging (MRI) and computed tomography (CT)). Image reconstruction is critically important: it takes the raw data from a scanner and represents it as a meaningful image to the user. However, an ongoing major challenge is the presence of noise in the raw data (especially in PET or SPECT), meaning that many possible images can represent the raw data.
Academics at King’s College London have developed a novel, self-supervised solution to image reconstruction, especially for PET or SPECT. The technology does not need a manufacturer or a user to specify the crucial parameters for reconstruction, which govern how to compensate for noise. By leveraging the raw data from a scan, the approach chooses for itself how to reconstruct an image to represent the raw data objectively. This also offers great help for multi-modal imaging (such as simultaneous PET-MR), where it is not known exactly how much prior information can be appropriately exploited.
Technology
Image reconstruction is essential for most imaging devices, including in medical imaging. These scanners deliver raw data, and image reconstruction takes that raw data and represents it as a meaningful image to the user, allowing the data to be exploited for tasks with far reaching applications. In medical imaging, the images enable understanding, diagnosing and staging of diseases (such as cancer, heart disease and brain disorders). In other imaging applications, the reconstructed images have utility in contexts such as security (security checks, satellite surveillance) and safety (non-destructive testing).
However, noise in the raw data means that many different image reconstructions are possible. There is therefore a burden of choice on the manufacturer or the user of imaging devices to decide how best to represent the raw data from a scan as an image. Furthermore, if information from other modalities is to be used (such as MR images to assist PET image reconstruction) there is again the issue of deciding how much to use the MR information to guide the reconstruction. Hence, when scanning a patient, the image which is presented to a clinician will in fact depend on how the manufacturer, or the user, chose to reconstruct the raw data as an image. Many different images could have been presented to the clinician as the representation of the scan data, and each one could be read differently, potentially leading to different medical assessments. Therefore much care is needed in choosing the parameters which determine which single image to use for interpretation purposes, particularly in the case of multi-modal image reconstruction. Objective image reconstruction remains an ongoing issue for the medical imaging industry.
The method developed by King’s College London inventors overcomes this problem by allowing the raw data to self-supervise and precisely choose for itself just how to reconstruct an image to objectively respresent the raw data. As an example, the images below from a simultaneous PET-MR scanner show how the method automatically and precisely selects the level of noise compensation for when MR information is used to compensate for highly noisy PET data.
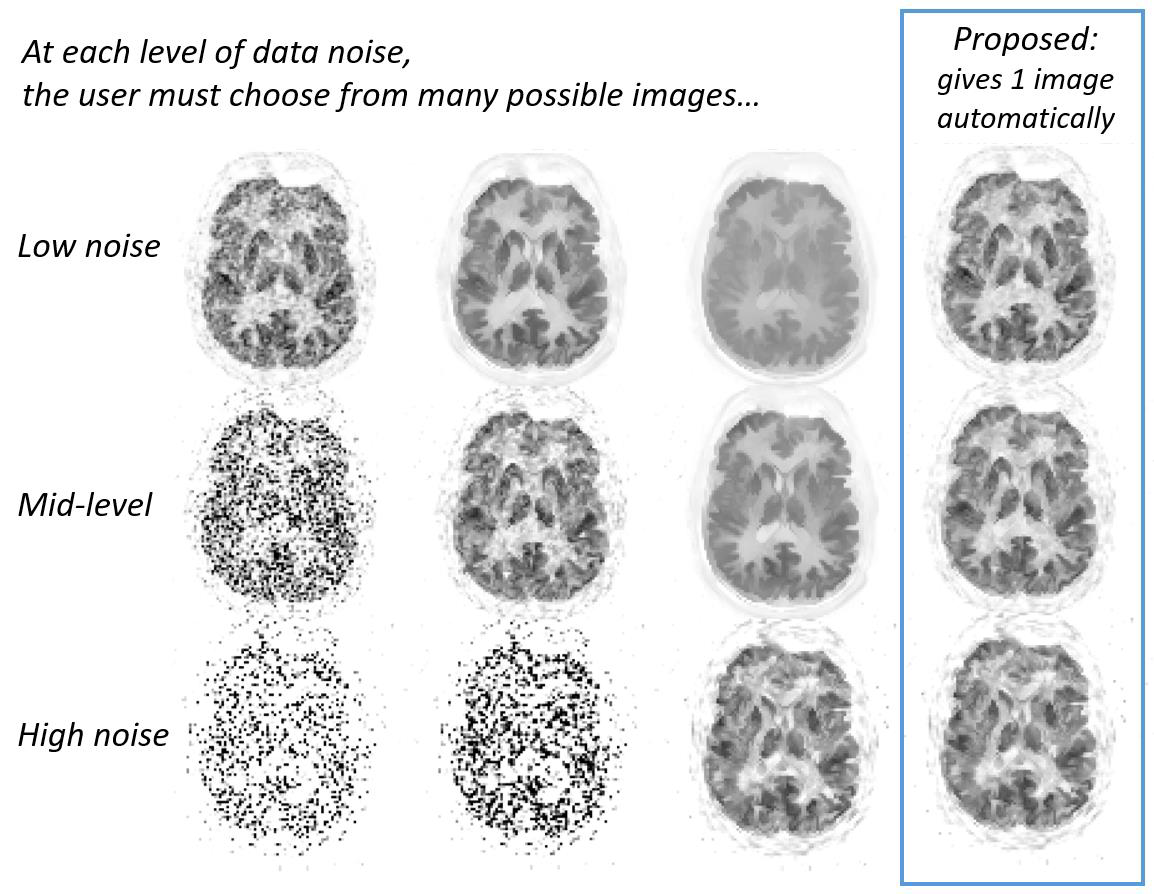
Benefits
• Confidence of image quality by automated selection of objective noise compensation.
• Immense scope for optimal use of any denoising method.
• Simplicity through a precise, automated and objective method.
• Theoretical appeal.
IP Status
A priority patent application has been filed in the UK (having priority date 03rd April 2019)
along with the PCT application-WO2020201755A1 (https://patents.google.com/patent/
WO2020201755A1/en?oq=PCT%2fGB2020%2f050876)